The goal of DataBytes is to share developments or new perspectives in the field of data science. These events often highlight work happening in industry to provide a pathway to connect industry with academia. We ask that presenters refrain from offering explicit sales or collaborative opportunities during or after the event. NCDS enjoys a close relationship with many higher education institutions, and we facilitate connections based on mutual interest. The NCDS offers other opportunities to provide thought leadership in a variety of platforms, please reach out to Amanda C. Miller to discuss this further or click below to navigate to the interest form.
Upcoming DataBytes Events
October 17, 12:00 - 1:00pm | Data Literacy 2025 and Beyond
About Veronica Blackburn
Veronica Blackburn is a seasoned leader in learning and development, with a rich background in enhancing data science education across higher education, government, and private sectors. As the Director of Learning at Elder Research, Veronica blends adult education best practices and complex analytics into practical teaching strategies. She also teaches mathematics and statistics at Northern Virginia Community College and holds a Master’s degree in Mathematics and Statistics from Georgetown University.
Past DataBytes Events | 2024
Using GenAI to win STTR and SBIR Government Grants | June 20, 2024
Through a competitive awards-based program, STTR and SBIR federal grants enable small businesses to explore their technological potential and open new opportunities to profit from its commercialization. However, it can be difficult for first-time applicants to get their foot in the door, between sorting through guidelines, requirements, and deadlines, and trying to locate successful examples. GrantScout is automating grant search, writing, and submission. The tool uses traditional deep learning methods and generative AI to unlock funding for everyone. Join GrantScout founders Felicia Chen and Jennifer Tang as they present how the government provides over two million grants for small businesses, the ways that their team fine-tunes their own models to create strong technical proposals, and lessons they’ve learned from past experiences that helped them build the platform.
Supporting AI Risk Management in the Analytics Lifecycle | April 30, 2024
The National Institute of Standards and Technology (NIST) released an AI Risk Management Framework for trustworthy and responsible use of AI and analytics. NIST offers a portfolio of measurements, standards, and legal metrology to provide recommendations that ensure traceability, enable quality assurance, and harmonize documentary standards and regulatory practices. Their framework is very detailed with recommendations across four functions: govern, map, measure, and manage. In this session, we’ll discuss incorporating these recommendations into the analytics lifecycle. Attendees to this session will gain a greater understanding of trustworthy AI best practices as well as user roles and expectations for building responsible analytics.
Join NCDS as Sophia Rowland, a Senior Product Manager focusing on ModelOps and MLOps at SAS, walks us through this important presentation.
View a recording of the event here.
Becoming A Data Detective - Holding AI Accountable | February 22, 2024
Bias and brittleness in artificial intelligence (Al) tools are a growing concern. Join Hilke Schellman, Emmy-award winning investigative reporter, Wall Street Journal and Guardian contributor and Journalism Professor at NYU, as she shares key takeaways from her book, The Algorithm: How Al Decides Who Gets Hired, Monitored, Promoted, and Fired and Why We Need to Fight Back Now.
Al is now being used to decide who has access to an education, who gets hired, who gets fired, and who receives a promotion. Algorithms are on the brink of dominating our lives and threaten our human future-if we don't fight back. During the webinar, Schellmann will share takeaways about the rise of Al in the world of work and show how she tested many of the available tools herself without coding experience.
During our time together, Hilke will share a few key takeaways from the book and answer questions from the audience. You don't want to miss this.
View a recording of the event here.
Past DataBytes Events | 2023
Causal AI: The Key to High-Stakes Decision Making | December 5, 2023
The National Consortium for Data Science looks forward to welcoming back Christopher Lam, CEO of Epistamai on December 5th for our next DataBytes event as he discusses Causal AI: The Key to High-Stakes Decision Making.
There has been tremendous attention to the generative AI wave and its enormous potential to transform industries. But there is a hidden wave developing right behind it called causal AI. Whereas generative AI is optimized for low-stakes decisions like chatbots and image generation, it is not designed to address issues like ethics or trustworthiness that are essential for using AI in high-stakes decisions like credit and hiring decisions. This is where causal AI fits into the picture.
In this presentation, Christopher Lam will discuss how to use causal AI to build AI systems that society can trust for high-stakes decision making. Lam will show how causal AI can help bridge the gap between symbolic AI and machine learning, demonstrating the value of integrating human knowledge and reasoning about the world to improve how data is analyzed. He will demonstrate through a use case how this more human-centric approach to AI can be used to build fairer and more equitable AI systems that are aligned with society's democratic values. Finally, he will describe a new causal hierarchy, one that integrates machine learning with causal inference and system dynamics.
View a recording of the event here.
The Risks of Facial Recognition Technology: Dismantling the First Amendment Defense | October 11, 2023
In a lawsuit challenging its surveillance activities, Clearview AI used the First Amendment as a defense. The facial recognition technology company argued that the creation and use of its surveillance product was First Amendment protected speech. Join Talya Whyte, third-year law student at New York University, as she presents a case study on the parties’ basic arguments, Clearview AI’s characterization of its activities as “speech,” and the implications of this argument. Attendees will understand how facial recognition technology works and the risks and harms inherent in its building and implementation, and gain the knowledge to make more informed legal, policy, and technical choices about the implementation of AI-based surveillance technology.
Talya Whyte is a third year law student at New York University. Her research interests lie at the intersection of new technology, society, public trust, and digital rights. She is a 2023 Google Legal Scholar, a Student Fellow at the Engelberg Center on Innovation Law & Policy, and NYU Cyber Scholar. Whyte hopes for a thoughtful and humanitarian integration of technology into existing legal and societal frameworks.
View a recording of the event here.
AI in Target Identification and Drug Discovery: Transforming the Future of Medicine | August 22, 2023
The National Consortium for Data Science looks forward to hosting Kimberly Robasky, Associate Director of Machine Learning/AI at Arrakis Therapeutics, on August 22 for our next DataBytes event as she discusses AI in Target Identification and Drug Discovery: Transforming the Future of Medicine.
Artificial intelligence (AI) is taking a transformative role in target identification and drug discovery. Today, AI algorithms can analyze vast, multi-modal datasets to identify drug targets, accelerate lead compound discovery, and optimize drug design. AI is being used by biotechnology companies around the world to compress timelines and improving clinical trial outcomes. Join us to uncover the data-driven revolution in personalized medicine enabled by AI-driven drug development.
View a recording of the event here.
AI Ethics Through the Lens of Causality with Chris Lam, founder and CEO of Epistamai | June 20, 2023
A Theory of Fairness
To understand fairness, one must unify central ideas from the social sciences and humanities to mathematics and computer science. In this talk, Chris will show how to model a principal cause of algorithmic bias (the structure vs. agency debate in sociology) and directly map it to the two fundamental laws of causal inference (counterfactuals/interventions vs. conditional independence). He will also show how to bridge the field of causal inference to machine learning, providing us with a novel way to visualize the different ways that a supervised machine learning model can discriminate. These causal models may help policymakers on both sides of the aisle to modernize AI regulations so that they are aligned to society’s values.
View a recording of the event here.
About the Speaker- Chris Lam
Chris is the founder and CEO of Epistamai, an AI research company based in the Research Triangle that is focused on understanding AI ethics through the lens of causality. The inspiration for his startup came from his work at the Federal Reserve, where he did research on algorithmic bias in credit decisions. He is an evangelist for the emerging field of causal data science, which could help us to solve intractable problems in data science today.
What does a Data Ethicist Do? With Anisha Nadkarni, Randstad Global | April 20, 2023
Data ethics is a growing concern in all industries, especially as issues such as algorithmic bias, informed consent, and privacy become more nuanced. Additionally, with artificial intelligence and machine learning tools gaining traction at a rapid speed, it is more imperative than ever that organizations establish strong ethical guidelines around the data collected from client projects, research endeavors, and business affairs. Anisha Nadkarni, Data Ethics Officer at Randstad Global, walks us through a day in the life of a data ethicist, with a Q&A session with Nadkarni at the end of the meeting.
Five Ways that Visualization Can Mislead (And How to Fix Them) with Dr. Danielle Szafir | February 14, 2023
Visualizations allow people to readily analyze and communicate data. However, many common visualization designs lead to engaging imagery but false conclusions. By understanding what people see when they look at a visualization, we can design visualizations that support more accurate data analysis and avoid unnecessary biases. UNC Computer Science Assistant Professor Danielle Szafir walks us through best practices in data visualization and analysis, with a Q&A session with Dr. Szafir at the end of the meeting.
View a recording of the event here.
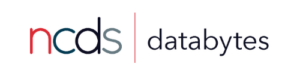